Kyle Wiggers* says researchers have come up with a new algorithm for tracking people in a densely packed crowd.
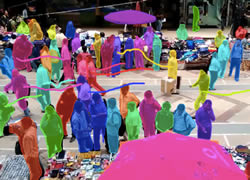
Image: Department of Computer Science, University of Maryland
Tracking dozens of people in dense public squares is a job to which AI is ideally suited if you ask scientists at the University of Maryland and University of North Carolina.
A team recently proposed a novel pedestrian-tracking algorithm — DensePeds — that’s able to keep tabs on folks in large crowds by predicting their movements, either from front-facing or elevated camera footage.
They claim that compared with prior tracking algorithms, their approach is up to 4.5 times faster and state-of-the-art in certain scenarios.
The researchers’ work is described in a paper (“ DensePeds: Pedestrian Tracking in Dense Crowds Using Front-RVO and Sparse Features ”) published last week on the preprint server Arxiv.org.
“Pedestrian tracking is the problem of maintaining the consistency in the temporal and spatial identity of a person in an image sequence or a crowd video,” the coauthors wrote.
“This is an important problem that helps us not only extract trajectory information from a crowd scene video but also helps us understand high-level pedestrian behaviours.”
As it turns out, tracking in dense crowds — i.e., crowds with two or more pedestrians per square metre — remains a challenge for AI models, which must contend with occlusion caused by people walking close to each other and crossing paths.
Most systems compute bounding boxes around each pedestrian, and problematically, these bounding boxes often overlap, affecting tracking accuracy.
In the pursuit of better performance, the team introduced a new motion model — Frontal Reciprocal Velocity Obstacles, or FRVO — which uses an elliptical approximation for each pedestrian and estimates position by considering things like side-stepping, shoulder-turning, and backpedalling, and collision-avoiding changes in velocity.
They combine it with an object detector that generates feature vectors (mathematical representations) by subtracting noisy backgrounds (i.e., pedestrians with significant overlap) from the original bounding boxes, effectively segmenting out pedestrians from their bounding boxes and reducing the likelihood that the system loses sight of any one of them.
To validate DenseNet, the researchers benchmarked it against the open source MOT dataset and a curated corpus of eight dense crowd videos chosen for their “challenging” and “realistic” views of crowds in public places.
They report that DensePeds produced the lowest false negatives of all baselines, and that in separate experiments which replaced the models with regular bounding boxes, it cut down on the number of false positives by 20.7 per cent.
* Kyle Wiggers is a technology journalist and AI correspondent for Venture Beat. He tweets at @Kyle_L_Wiggers and his website is kylewiggers.com.
This article first appeared at venturebeat.com.