Peter Bright* reports that problem with relying on an algorithm to develop facial recognition technology is that it can create a prejudiced system.
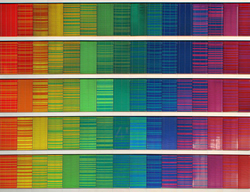
Photo:Ricardo Gomez Angel
Microsoft has improved its facial recognition system to make it much better at recognising people who aren’t white and aren’t male.
The company says that the changes it has made have reduced error rates for those with darker skin by up to 20 times and for women of all skin colours by nine times.
As a result, the company says that accuracy differences between the various demographics are significantly reduced.
Microsoft’s face service can look at photographs of people and make inferences about their age, gender, emotion, and various other features; it can also be used to find people who look similar to a given face or identify a new photograph against a known list of people.
It was found that the system was better at recognising the gender of white faces, and more generally, it was best at recognising features of white men and worst with dark-skinned women.
This isn’t unique to Microsoft’s system, either; in 2015, Google’s Photos app classified black people as gorillas.
Machine-learning systems are trained by feeding a load of pre-classified data into a neural network of some kind.
This data has known properties — this is a white man, this is a black woman, and so on — and the network learns how to identify those properties.
Once trained, the neural net can then be used to classify images it has never previously seen.
The problem that Microsoft, and indeed the rest of the industry, has faced is that these machine learning systems can only learn from what they’ve seen.
If the training data is heavily skewed toward white men, the resulting recogniser may be great at identifying other white men but useless at recognising anyone outside that particular demographic.
This problem is likely exacerbated by the demographics of the tech industry itself: women are significantly underrepresented, and the workforce is largely white or Asian.
This means that even glaring problems can be overlooked — if there aren’t many women or people with dark skin in the workplace, then informal internal testing probably won’t be faced with these “difficult” cases.
This situation produces systems that are biased: they tend to be strongest at matching the people who built them and worse at everyone else.
The bias isn’t deliberate, but it underscores how deferring to “an algorithm” doesn’t mean that a system is free from prejudice or “fair.”
If care isn’t taken to address these problems up front, machine learning systems can reflect all same biases and inequalities of their developers.
Microsoft’s response was in three parts.
First, the company expanded the diversity of both its training data and the benchmark data used to test and evaluate each neural network to see how well it performs.
This means that the recogniser has a better idea of what non-white non-men look like and that recognisers that are weak at identifying those demographics are less likely to be selected.
Second, Microsoft is embarking on a new data collection effort to build an even broader set of training data, with much greater focus on ensuring that there’s sufficient diversity of age, skin colour, and gender.
Finally, the image classifier itself was tuned to improve its performance.
The company is also working more broadly to detect bias and ensure that its machine learning systems are fairer.
This means giving greater consideration to bias concerns even at the outset of a project, different strategies for internal testing, and new approaches to data collection.
* Peter Bright is Technology Editor at Ars Technica in Brooklyn, USA and he tweets at @drpizza.
This article first appeared at arstechnica.com.